AI has huge potential in banks—from automating operations to uncovering customer insights, enhancing compliance, and streamlining everything from KYC to collateral management.
But how do you actually deploy it?
At Finaumate, we’ve worked closely with financial institutions navigating this journey. Here’s our take on the eight key elements that make the difference between AI pilots that stall—and those that scale. The question is not only if you are ready for your transformation as analysed HERE, but also what to do.
Key Takeaways
✅ 1. Start Small, But Start Smart
AI success in banking begins by choosing the right use cases—those that are impactful, feasible, and aligned with business goals. Focus on solving real problems, not just experimenting with technology.
✅ 2. Strong Foundations Matter
High-quality data, scalable infrastructure, and cross-functional teams are critical for AI to deliver value. Without these core elements, even the best models will fail to perform in the real world.
✅ 3. Governance and Change Are Just as Important as Tech
Effective AI deployment isn’t just a technical challenge—it’s an organizational one. Build clear governance, engage your risk and compliance teams early, and invest in change management to bring people along.
AI is reshaping finance and banking – don’t get left behind. Our exclusive newsletter delivers insights you simply can’t afford to ignore. Subscribe HERE.
1. Start with the Right Use Cases
Before jumping into tools and models, start by aligning AI with your business strategy. What are the pain points? Where can AI really add value to a bank? We advise banks to begin with high-impact, low-resistance areas—like automating back-office processes (think reconciliations or trade matching), or helping teams make faster, data-backed decisions.
Don’t try to do everything at once. Prioritize based on feasibility and business impact. We use a simple 2×2 matrix to help clients identify where Artificial Intelligence can generate the most value quickly.
2. Build a Strong Data Foundation for AI in banks
No matter how good your model is, if your data is messy or siloed, your AI will struggle.
You’ll need high-quality, well-governed, accessible data. That means investing in data infrastructure, ideally with cloud capabilities, clean data pipelines, and clear ownership. Data governance should be front and center: define who owns what, ensure compliance (especially around client data), and get serious about quality checks.
We often see successful AI initiatives start with a parallel data-cleaning sprint. It’s not glamorous, but it’s essential.
3. Choose Scalable Infrastructure
When you’re deploying Artificial Intelligence in banking, you can’t afford to play around with infrastructure that can’t scale or comply.
We typically recommend cloud-based or hybrid solutions that are secure, scalable, and flexible enough to support AI workloads. You’ll want architecture that allows fast experimentation and model updates, with strong controls around data handling and auditability.
We also guide clients to integrate these systems with their existing tech stacks rather than replacing everything overnight.
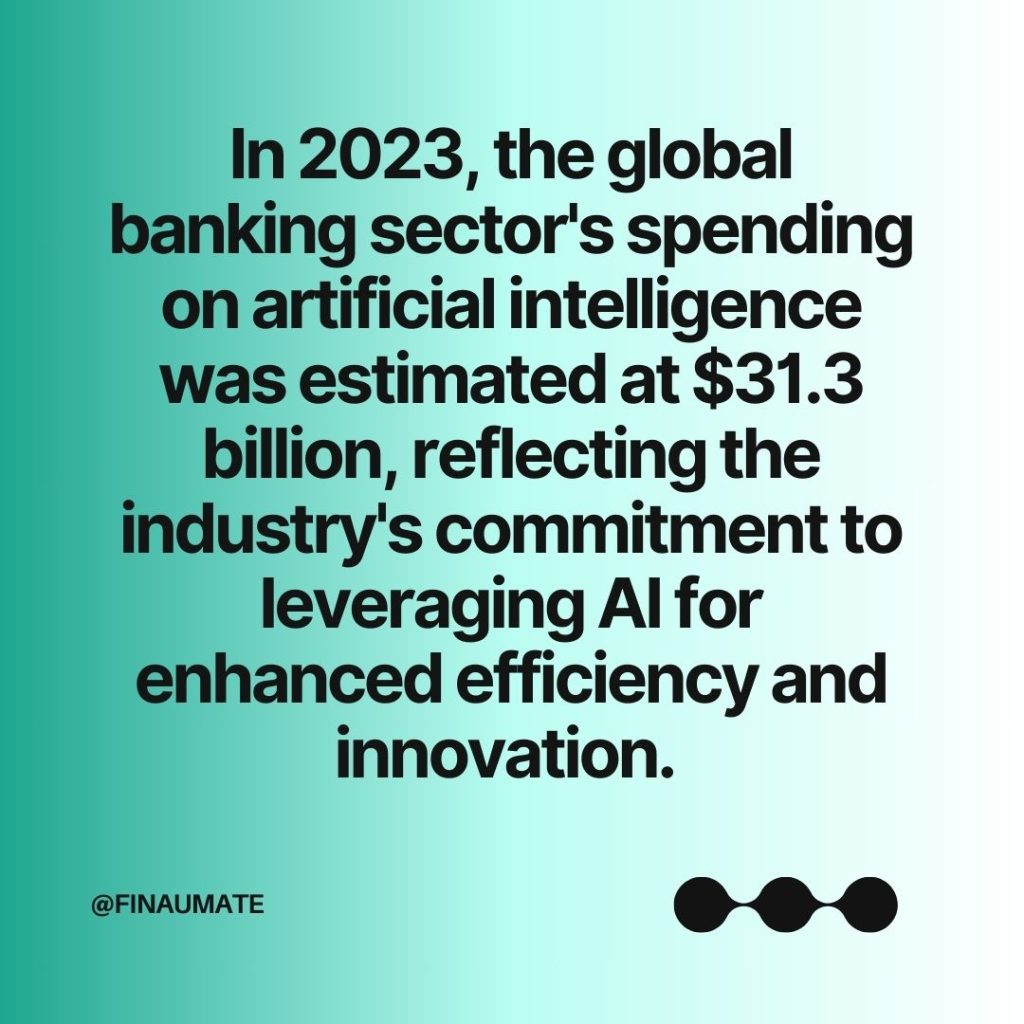
4. Assemble the Right Team for your AI project
AI success isn’t just about hiring data scientists. You need a team that includes operations experts, compliance officers, engineers, and business owners—all working together.
In our experience, the best teams are cross-functional and co-located (even virtually). They test fast, get feedback, and work in sprints. And they understand that deploying AI in banking isn’t just about technology—it’s about solving problems that matter to the business.
For your AI project in your bank and if you’re hiring, look for people who can bridge the technical and the practical.
5. Set Up Governance Early
Artificial Intelligence without governance is a recipe for risk. But too much governance early on can also kill innovation.
We recommend a phased approach: start with light-touch governance for exploration, but make sure you build a framework for model validation, risk controls, explainability, and regulatory compliance as you scale. And yes—this means working closely with your risk and legal teams from day one.
We’ve helped several banks set up governance models that evolve with maturity. It’s about balance: enough structure to manage risk, not so much that you block progress.
6. Invest in Change Management
AI doesn’t just change tools—it changes workflows, decision-making, and how teams operate.
To make it work, you’ll need a change plan. That includes training employees, demystifying AI, and making sure business users feel involved—not threatened.
We often run internal “AI discovery” workshops with clients, helping teams understand what AI can (and can’t) do, and how it fits into their roles. People support what they help build.
7. Track Performance and Iterate
Don’t assume your AI model is done once it’s live. Real-world performance can drift, and business needs change.
Set up metrics and feedback loops from the start. Monitor your models continuously. In many banks, we help set up internal AI “health checks” every quarter—looking at model accuracy, business value, and user feedback. These checkpoints help spot issues early and keep your AI aligned with evolving goals.
8. Collaborate Outside the Bank
Effectively, for your AI banking program, you don’t need to do everything in-house. In fact, you shouldn’t.
We’ve seen the best results when banks partner with AI specialists, cloud providers, regtech startups, and even academics. These collaborations speed up innovation and help you avoid blind spots. Our role often includes acting as the connector—bringing in the right tech partners while making sure everything fits into your governance and infrastructure.
Final Thought – AI in Banking
Deploying AI in banking is more than a tech project. It’s an evolution in how your institution operates, makes decisions, and serves clients. Make sure you know the challenges you will face in advance as described HERE, and work towards an effective solution.
Done right, it unlocks enormous value. But success depends on getting the fundamentals in place: the right data, the right use cases, the right team, and the right governance.
If you’re planning your next step with AI, we’re here to help.
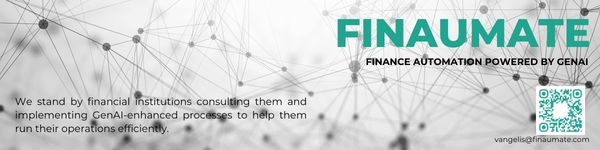