Governance is supposed to protect financial institutions — but when applied incorrectly to GenAI, it can stall progress and even kill innovation. In our previous article, we explored why financial services struggle to scale GenAI. One key reason is governance.
Financial institutions face three major governance challenges when it comes to deploying GenAI, particularly around inference infrastructure. Let’s explore these challenges — and how to fix them.
GenAI is reshaping finance and banking – don’t get left behind. Our exclusive newsletter delivers insights you simply can’t afford to ignore. Subscribe HERE.
Key Takeaways
1️⃣ Use on-premise setups to ensure data protection and simplify compliance.
2️⃣ Set guardrails at the application level to avoid limiting model performance.
3️⃣ Improve data access with secure sandbox environments to accelerate GenAI development.
1. Data Protection and Data Sovereignty
Financial institutions take data protection seriously — and for good reason. However, using cloud-based LLM (Large Language Model) setups or models as a service (like OpenAI) introduces serious risks. If sensitive client data is sent through a prompt, it could end up in the wrong hands.
The challenge is that ensuring data security in cloud setups imposes a heavy burden on compliance and legal teams. Governance reviews and security checks create bottlenecks that slow down deployment and increase costs.
Many companies attempt to solve this with PII (Personally Identifiable Information) filters — but this often backfires. PII filters make the model less effective by stripping out valuable context. Even if the model works, the inability to process sensitive data reduces the value GenAI can provide.
✅ Solution:
The best way to address this challenge is by using on-premise installations. On-premise setups give you full control over your model endpoint and data, ensuring that sensitive information remains secure. By creating localized endpoints, institutions can maintain data sovereignty and reduce compliance burdens.
2. Cybersecurity
Cybersecurity presents a major challenge when using cloud-based LLM setups. Third-party security reviews are time-consuming, and connecting to external parties creates new vulnerabilities that need to be identified and resolved before deployment.
Even with on-premise installations, cybersecurity teams often try to impose guardrails at the inference level to prevent issues like hallucinations and prompt injections. This is misguided. Guardrails should be applied at the application level, not at the inference point.
When guardrails are imposed at the inference level, they can restrict model performance and make the system unusable for most real-world applications. For example, overly aggressive content filtering may prevent the model from generating accurate or contextually useful outputs.
✅ Solution:
Instead of imposing broad guardrails at the inference level, financial institutions should apply guardrails at the application level — specific to each use case. This ensures that the model remains effective while addressing cybersecurity risks where they matter most.
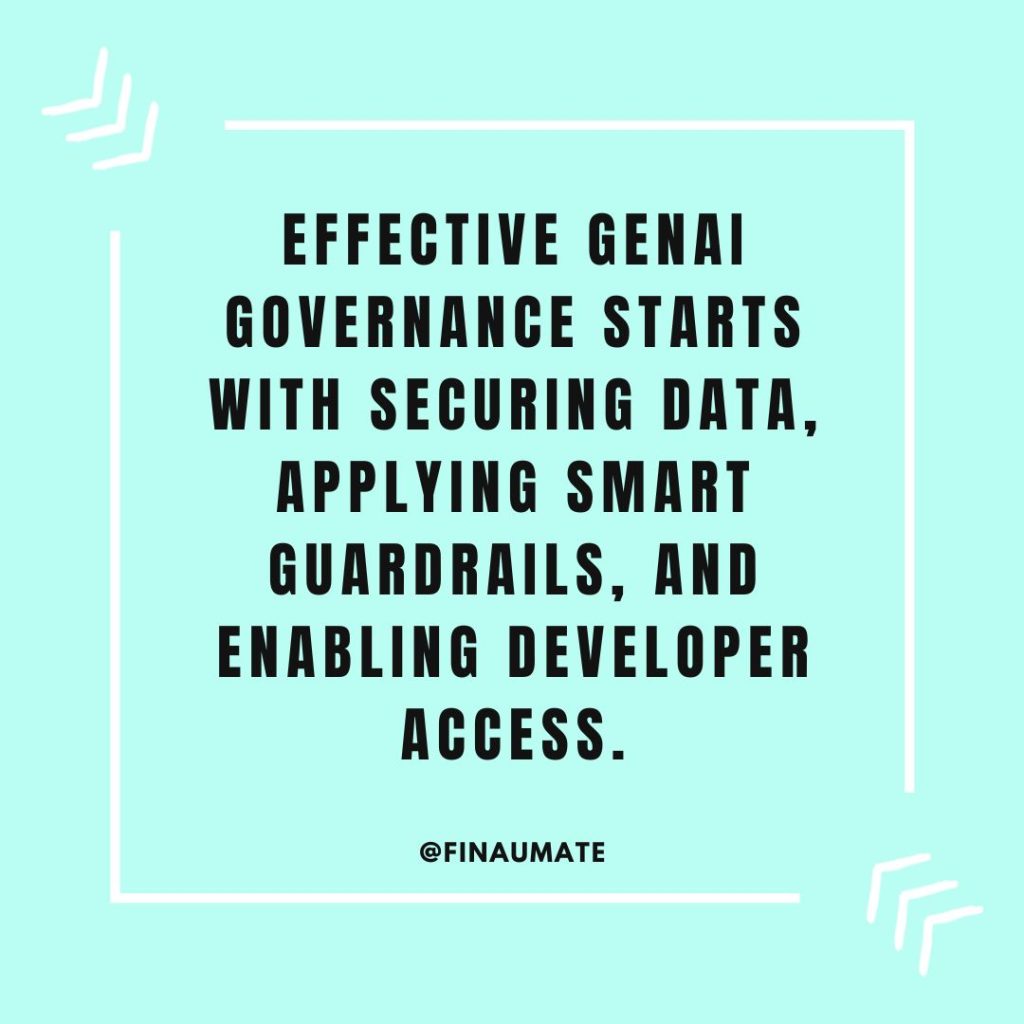
3. Data Access
Data access is often restricted on a need-to-know basis, which makes sense for security — but it creates major barriers for AI developers. Without sufficient access to data, developers can’t train and refine GenAI models effectively.
Strict data access policies cause delays and limit the value GenAI can deliver. Data access requests often face long review cycles, and in many cases, developers are denied access altogether. Additionally, the lack of appropriate sandbox environments limits the ability to experiment and improve model performance.
✅ Solution:
Financial institutions need to:
- Revisit data access policies to give AI developers appropriate access to data while maintaining security.
- Create secure sandbox environments where developers can safely work with data without exposing it to broader risks.
By improving data access policies and providing the right development environments, financial institutions can accelerate GenAI deployment and improve model quality.
Our Conclusions and Thoughts
While many stakeholders are looking into solutions how AI supports financial institutions and banks to tackle compliance in the market (a very well related article from McKinsey can be read HERE), the same firms ignore to work on their own governance issues. To unlock the full potential of GenAI in financial services, institutions need to rethink their governance models. On-premise installations, application-level guardrails, and more flexible data access policies will remove bottlenecks and enable faster, more secure GenAI deployment.
Governance should enable innovation — not block it. By adjusting governance frameworks to fit the specific needs of GenAI, financial institutions can unlock new levels of efficiency, insight, and customer value.
🚀 Need Help with GenAI Deployment?
At Finaumate, we specialize in AI infrastructure consulting for financial institutions. If you’re navigating the complexities of GenAI deployment, let’s talk! Contact us today to explore the best infrastructure strategy for your business.
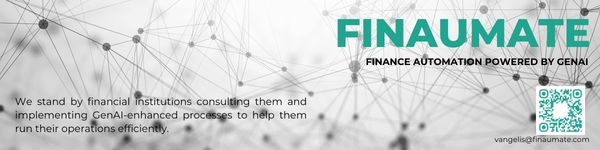