If you work in a major financial institution and find yourself wondering why GenAI in finance hasn’t made it to your company desktop, you’re not alone. Despite the hype around GenAI in finance, many large banks, insurers, and asset managers have yet to adopt these tools broadly. In fact, harnessing GenAI in finance for everyday tasks remains a distant ambition for countless regulated organizations.
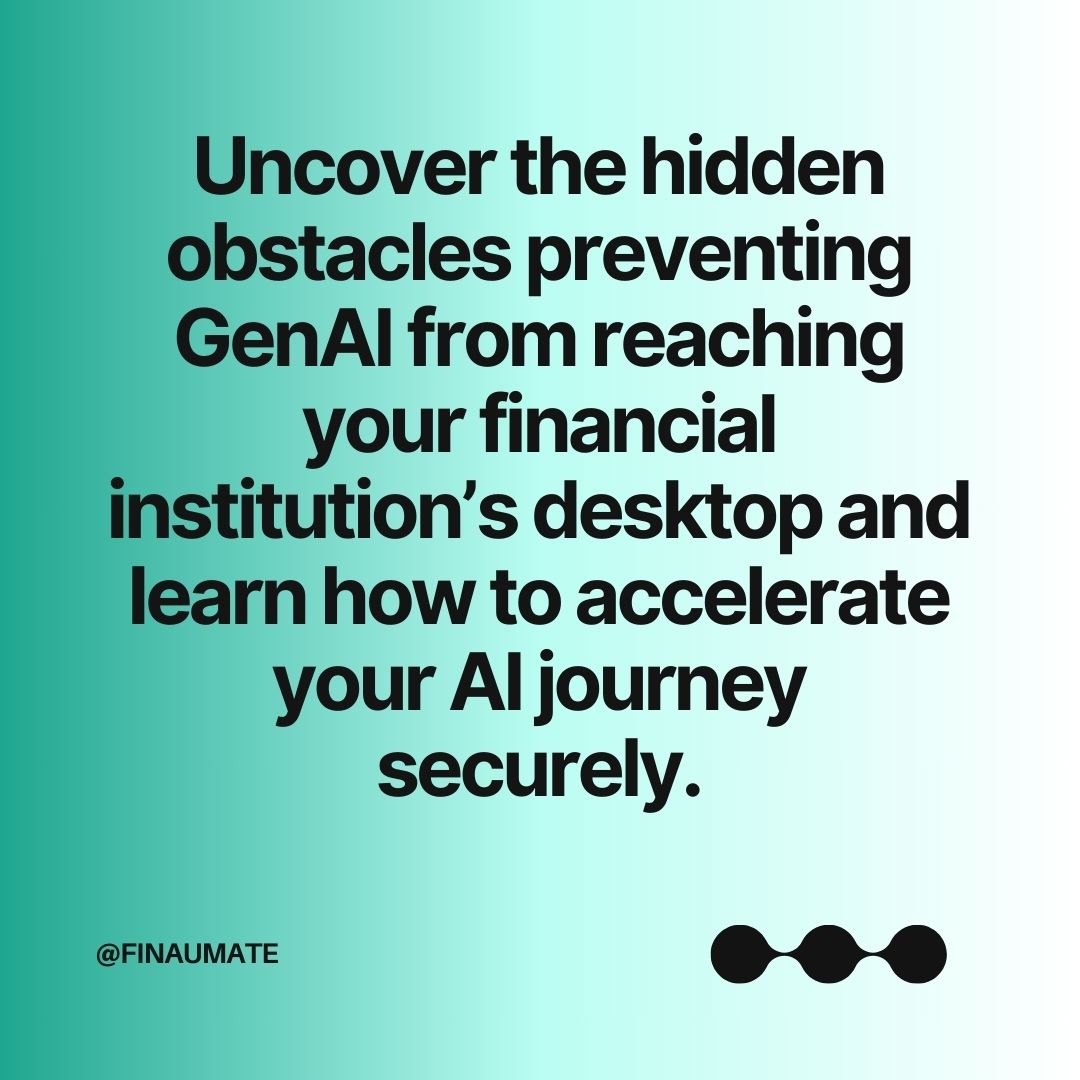
GenAI is reshaping finance and banking – don’t get left behind. Our exclusive newsletter delivers insights you simply can’t afford to ignore. Subscribe HERE.
Costs: Why Scaling GenAI Doesn’t Come Cheap
Although many sources are supportive of the current trend like this one published by UKFinance, one of the biggest reasons organizations in regulated industries delay rolling out desktop GenAI solutions is the hefty cost of scaling. Unlike traditional software, LLM solutions (Large Language Models) demand enormous computing resources. Cloud-based AI services often require multiple times the typical infrastructure budget for hardware, networking, and software licensing—leading to significant AI infrastructure costs. For financial institutions governed by strict spending oversight, justifying these costs isn’t simple.
Expertise: Finding Skilled AI Teams
High costs aren’t the only hurdle. Even if you can afford the technological investment, you still need specialized talent. AI adoption in banks involves more than just clicking “deploy.” Your team must be well-versed in enterprise-scale AI deployment, from compliance monitoring systems to AI risk management. You can outsource some knowledge to cloud providers, but you still need in-house professionals who understand NLP (Natural Language Processing) models, data protection, and overall AI integration challenges. This skill gap often delays projects for months—or even years.
Data Privacy and Confidentiality: No Margin for Error
In compliance in regulated industries, any oversight can be catastrophic. A single misstep where an employee inadvertently shares sensitive data with a chatbot could land your organization in hot water. While guardrails and filters exist to prevent such issues, they can be overly aggressive or too lenient. This makes many financial firms—particularly those that handle critical customer details—hesitant to roll out large-scale financial services automation using public ChatGPT alternatives or Microsoft Copilot in finance. The bottom line: data privacy and confidentiality cannot be compromised.
Private Hosting vs. Cloud: Weighing Your Options
For some, private cloud AI or even on-premise AI hosting appears as a solution to tighten security controls. However, self-hosting large-scale AI systems comes with its own burdens. The hardware investment alone can be enormous, and data centers often deal with a shortage of rack space. Scaling for AI inference (the process of generating responses from a pre-trained model) can be feasible, but training or fine-tuning LLMs in-house may demand more computing power—and time—than many institutions can manage. And since large financial firms typically have lengthy hardware procurement processes, your AI-powered productivity timeline might stretch much further than you’d like.
Why Regulated Industries in Finance Lag Behind in the GenAI domain
Companies outside finance can sometimes adopt generative AI in a matter of weeks. But for banks, insurers, and asset managers, launching new technology involves an intricate dance among multiple stakeholders—IT security, compliance, risk management, legal, and beyond. Even a pilot phase may face numerous approvals and security reviews. In many cases, the fear of non-compliance overshadows the allure of cutting-edge tech, delaying a broad rollout of GenAI in finance on employee desktops.
Looking Ahead: How to Clear the Path in Finance with GenAI
Despite these hurdles, you can still make progress with a well-planned strategy:
- Start Small: Begin with a controlled proof of concept focusing on a single business function where GenAI tools for insurers or bankers can truly add value.
- Educate Stakeholders: Host internal workshops or training sessions. Foster understanding among compliance, legal, and IT teams so they feel comfortable with the technology’s capabilities.
- Partner Wisely: If you lack internal AI pros, consider outside experts who specialize in enterprise-scale AI deployment for regulated industries.
- Plan for Compliance: Build robust guardrails for data handling, encryption, and monitoring. A bulletproof data privacy framework can alleviate the biggest concerns early on.
Conclusion: Take the Next Step with Finaumate
In a recent article we analyzed a related topic why GenAI failed so far in finance. So, why hasn’t GenAI reached your desktop yet if you are in finance? High costs, limited expertise, stringent data confidentiality rules, and sluggish hardware deployments all slow adoption in the financial sector. But these obstacles aren’t permanent. By carefully addressing each issue and crafting a thoughtful roadmap, you can eventually unlock the enormous potential of AI for your financial institution.
Ready to accelerate your AI journey? Visit us at Finaumate and discover how we can guide you toward safe, compliant, and scalable GenAI solutions for your bank, asset management firm, or insurance company.
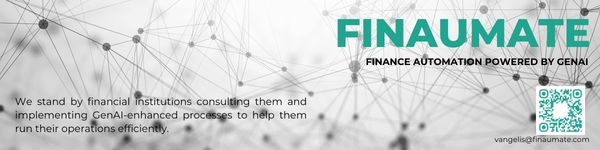