Every leadership team in financial services is asking the same question: How advanced are we in our AI capabilities compared to our peers?
That’s the essence of an AI capability assessment. Yet, most internal reviews rely too heavily on public benchmarks, glowing thought-leadership content, or industry rankings. These are helpful for marketing optics, but they can be dangerously misleading. Why? Because behind the headlines, many institutions are still struggling to move past pilot projects and internal red tape.
So how can you get an honest, actionable view of your organization’s AI readiness?
It is not just wondering how your governance can impact the AI deployment as we wrote HERE. It is also breaking down into these five simple but critical questions. Ask these, and you’ll get a much clearer view of where you stand—factually.
🔑 Key Takeaways: How to Perform an Effective AI Capability Assessment
Slow internal processes are a major risk to AI adoption
If AI teams face long delays in data access or use-case approvals, innovation stalls. Organizations must streamline workflows and governance to stay competitive in enterprise AI.
Public AI maturity rankings can be misleading
Don’t rely solely on industry surveys or press releases. A true AI capability assessment requires asking internal, fact-based questions about infrastructure, governance, and tool usability.
Assess your enterprise AI readiness with five essential questions
Evaluate access to large language models (LLMs), GPU infrastructure, productivity tools like MS Copilot, and approval processes for data and use cases. These metrics provide a realistic picture of AI readiness.
1. Do You Have Secure, Cloud-Based Access to Large Language Models?
Cloud access to LLMs like OpenAI’s GPT-4, Anthropic’s Claude, or open-source models on Azure and AWS is essential. But here’s the kicker: Can you use them with confidential or private data?
Many teams believe they have access, but it’s often in a read-only demo environment or disconnected from sensitive internal data.
Your AI capability assessment must ask:
- Is LLM access approved for internal data use?
- Can this access connect to your enterprise data sources, securely?
- Is data flow monitored, audited, and compliant with data protection standards?
💡 Best-in-class organizations have production-grade integrations via platforms like Azure OpenAI or Amazon Bedrock, with clear governance over how models interact with enterprise data.
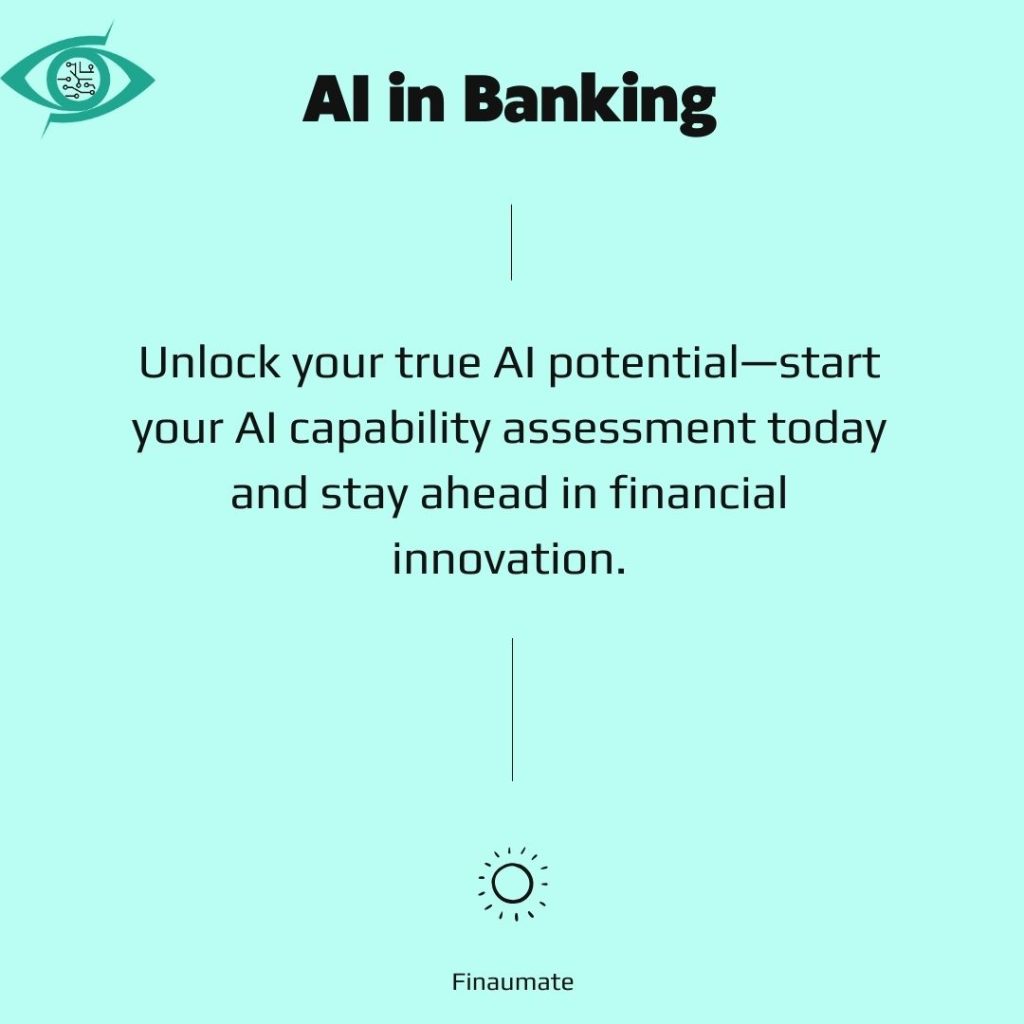
2. Do You Have On-Premise GPU Infrastructure for LLM Inference?
Not every use case can (or should) go to the cloud. For latency-sensitive or highly regulated AI workloads, on-premise GPU infrastructure is non-negotiable.
As we suggest, ask yourself:
- Do we have dedicated infrastructure for LLM inference?
- What’s the total available GPU VRAM? (A strong benchmark: 640 GB minimum.)
- Is this capacity accessible in sandbox or production environments, with secure access to live data?
⚙️ Example: Leading banks are investing in NVIDIA H100 clusters to bring model inference closer to their data. Goldman Sachs, for instance, has been vocal about its private AI infrastructure investments.
3. Are AI Productivity Tools Actually Useable for Your Staff?
This one’s trickier than it seems. Yes, you may have licenses for MS Copilot, ChatGPT Enterprise, or other AI productivity tools. But are they truly usable on the desktop?
I’ve seen cases where tools were technically “available,” but locked down by so many internal guardrails they became frustrating to use.
Questions to ask:
- Can non-technical staff use generative AI tools without IT support?
- Are there limitations that make them unusable in day-to-day tasks?
✨ Tip: A good test is whether staff in operations or legal teams are actively using AI tools without needing workaround hacks.
4. How Long Does It Take to Get Data Access Clearance for AI Assessment?
If your AI developers are waiting weeks—or months—for access to training data, you’ve got a bottleneck.
However, A strong AI capability assessment includes:
- Time-to-data-access as a metric (aim for <2 weeks).
- Clearly defined roles and responsibilities for access approval.
- An automated process through data governance tools, not endless email chains.
5. To Assess AI; How Fast Can Use Cases Get Approval from AI Committees?
Here’s where great ideas go to die: committee hell.
Every AI project should be vetted—but if approval processes are too slow or opaque, you risk missing the window for innovation.
You should track:
- Time from idea submission to use case go-live.
- Frequency of committee reviews.
- Are business-line stakeholders empowered to escalate or fast-track?
📈 Pro tip: Organizations with agile governance models run lean AI councils that meet weekly, not quarterly.
Where Should You Be Today?
If you’re serious about not falling behind, benchmark yourself against these targets:
✅ You should fully satisfy either Question 1 or 2 (ideally both).
✅ A clear “yes” should be In Question 3 — and users should actually be using the tools.
✅ When you are looking at Questions 4 & 5 should be under 2 weeks, end-to-end.
If these don’t hold, you are at real risk of falling behind—no matter what your PR or internal reporting says.
Final Thoughts: Time for a Real AI Capability Assessment
Consequently, true AI maturity doesn’t lie in press releases or shiny demos. It lies in how usable, scalable, and secure your AI stack is—and how quickly your people can get things done.
So ask the right questions. Get the answers. And don’t settle for “we’ve got it covered” until you’ve verified it yourself.
🚀 Need help benchmarking or conducting an independent AI assessment? At Finaumate, we help financial institutions cut through the noise and build real AI capabilities. Get in touch with us today.
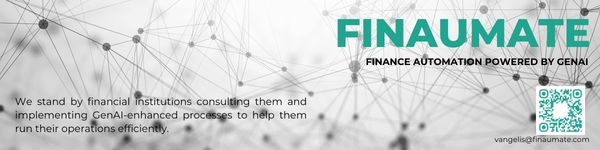